The pharmaceutical industry operates under one of the highest failure rates of any business sector. The success rate for drug candidates entering capital Phase 1 trials—the earliest type of clinical testing, which can take 6 to 7 years—is anywhere between 9% and 12%, depending on the year, with costs to bring a drug from discovery to market ranging from $1.5 billion to $2.5 billion, according to Science.
This skewed balance sheet drives the pharmaceutical industry’s search for machine learning (ML) and AI solutions. The industry lags behind many other sectors in digitization and adopting AI, but the cost of failure—estimated at 60% of all R&D costs, according to Drug Discovery Today—is an important driver for companies looking to use technology to get drugs to market, says Vipin Gopal, former chief data and analytics officer at pharmaceutical giant Eli Lilly, currently serving a similar role at another Fortune 20 company.
“All of these drugs fail due to certain reasons—they do not meet the criteria that we expected them to meet along some points in that clinical trial cycle,” he says. “What if we could identify them earlier, without having to go through multiple phases of clinical trials and then discover, ‘Hey, that doesn’t work.’”
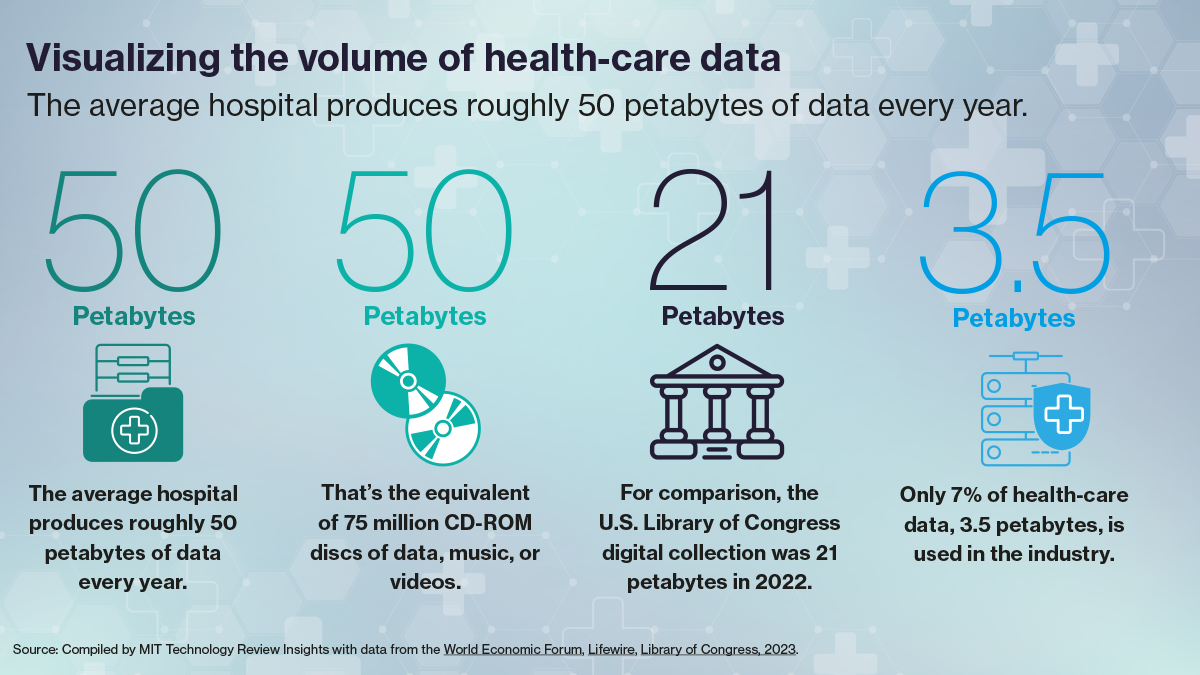
The speed and accuracy of AI can give researchers the ability to quickly identify what will work and what will not, Gopal says. “That’s where the large AI computational models could help predict properties of molecules to a high level of accuracy—to discover molecules that might not otherwise be considered, and to weed out those molecules that, we’ve seen, eventually do not succeed,” he says.
This content was produced by Insights, the custom content arm of MIT Technology Review. It was not written by MIT Technology Review’s editorial staff.
Article Source link and Credit